Whether for editing and translating, for drafting and summarising, for creating images or reviewing papers, Artificial Intelligence softwares and generative tools are increaslingly being used in research.
When it comes, more specifically, to research data and their management, two important considerations must always be kept in mind:
1) Individuals are at the core of research processes; thus, always make sure to attain to an ethical, legally-compliant and conscious use of Artificial Intelligence tools. This is the reason why UNIMI has promoted 10 Principles for Governing Artificial Intelligence within the University.
2) Garbage in, garbage out (GIGO)
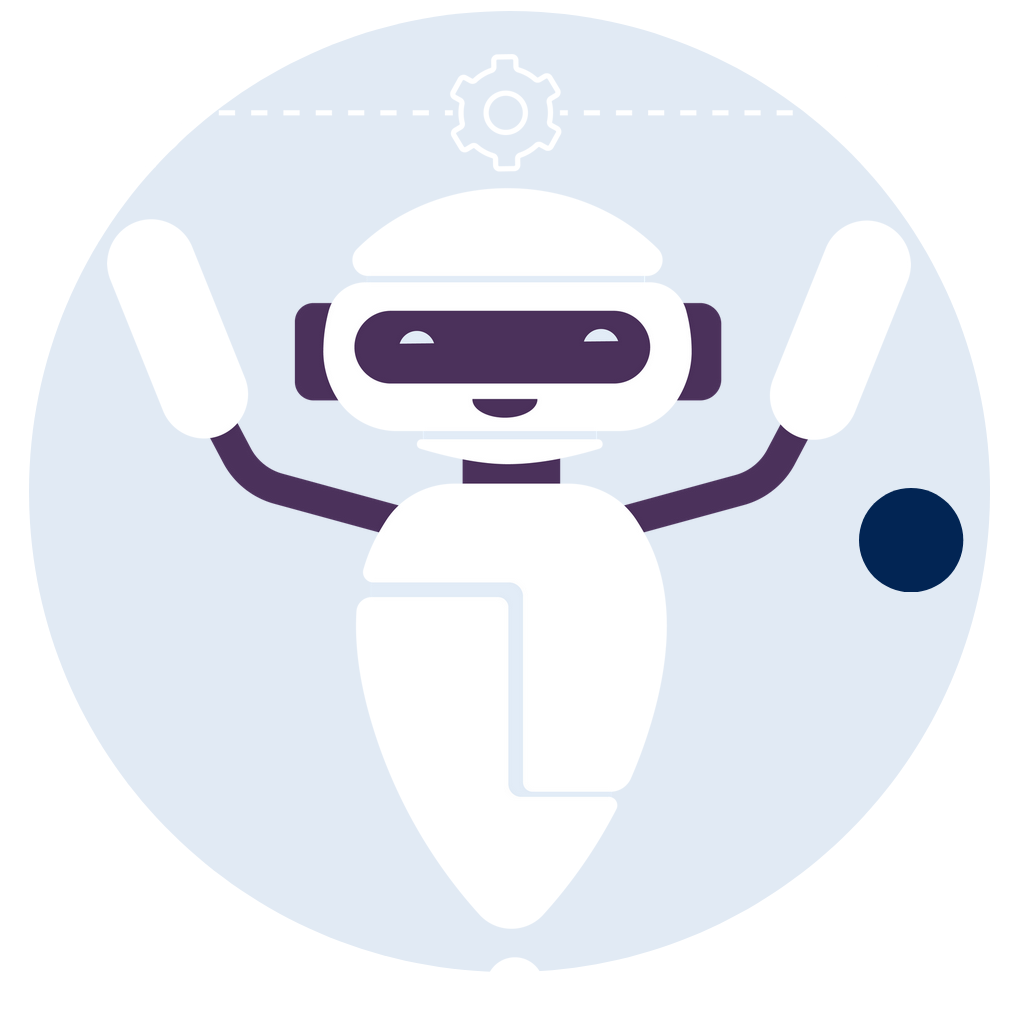
What does GIGO mean? "Data is the foundation of any AI system, no matter how complex or performant the system. Since AI models are designed to take in data, process it, and then make decisions or predictions based on that data, the quality of the data is critical to the accuracy and reliability of the model. Poor quality data can lead to incorrect results or negative outcomes, while high quality data can provide actionable insights and meaningful predictions" (see saifr blog). Thus, biased or poor quality data taken as an input may result in an ouput of similarly scarce quality.
How to face it? As reported in UNIMI's 10 principles, the standard of data minimisation requires users to exercise special caution when entering information into AI tools, and to only enter the information necessary to achieve the intended purpose. Thus, always pay attention to the kind of data (and its quality) when you are consciously using a generative AI system. But what if you openly share your data on a research data repository and this is taken as an input by a generative AI system automatically, without your knowledge?
This is precisely why open data by itself is not enough: open data should always also be FAIR data
To do this, your data must be accompanied by clear, detailed, contextualized information about data collection, analysis and elaboration; and, importantly, data quality should be guaranteed too. You should also ensure not to provide data which is inaccurate or incomplete, which is poorly structured (since it may be misleading), and, in particualr, data which is biased.
Useful resources:
- EU Artificial Intelligence Act, article 10: Data
- Watch UNIMI's seminar on AI and Open scientific research
- Take Nature’s AI research test: find out how your ethics compare
- AI ready data
- Mitigating risks and maximizing benefits of AI in research
- FAIR-R principles: advancing AI ready data